Win for FEA at ORE Catapult & Scottish Power Hackathon
With the ever-increasing share of wind generation on the GB system, National Grid ESO and power generators face an increasingly difficult challenge: How to integrate wind capacity to its full potential. Knowing when wind assets can be dispatched after curtailment or when they can provide frequency response is challenging if you don’t have an accurate picture of a sites instantaneous potential. In October 2019 the Offshore Renewables Catapult, in collaboration with Scottish Power and National Grid, ran an open competition to address precisely this challenge.
The aim was to bring together teams from the private sector and academia with experience in data science and artificial intelligence to create higher accuracy predictions of power available using SCADA data and state of the art machine learning approaches. Teams were provided with six months of data for a Scottish Power onshore windfarm of 16 turbines (37 MW) and given 36 hours to build and test models before being given the evaluation data. Achieving the highest accuracy out of all the teams (0.55 Mean Absolute Error), Future Energy Associates (FEA) were delighted to win the competition and take home the £10,000 prize.
Our Approach:
Early research identified the location of the site and subsequently the technical specifications of the turbines (including the manufacturer, generation capacities and power curves), as well as geodata for the site. With limited SCADA data available (wind speed, generator speed, blade angle) additional measures of apparent power and wind direction were generated to add to the models. From the irregularly sampled SCADA data we sought to keep the highest resolution possible - analysis of frequency suggested a 10 second resampling rate would retain the most information whilst avoiding the need for too much interpolation.
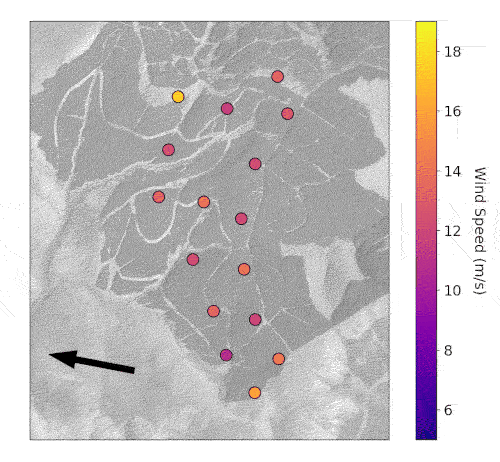
After exploratory analysis of the data, a combined bottom-up (turbine level) and a top-down (site level) approach was tested. The site level approach explored autoregressive moving average models (ARIMAX) and random forest algorithm based on site level SCADA data and apparent power, whilst the bottom-up approach tested neural networks, gaussian processes and gradient boosted random forests. In order to speed up the data processing an additional curtailment classifier was created using kernel density estimation, this was then added as a feature into the site level model.
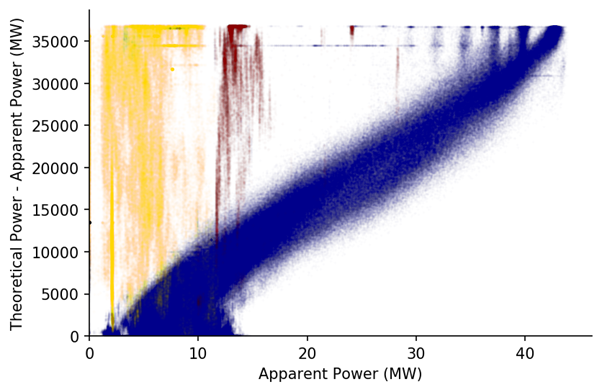
Keys to Success:
With many of the entrants employing similar classes of machine learning models, particularly neural networks and random forests, there are some more subtle differences in the FEA approach that gave our improved accuracy. Firstly, with only 30 minutes allowed to evaluate the final models, a key element of the approach was having a robust pipeline to clean and process the data to produce final predictions. Using a higher resolution of sampling in this process was likely a strong contributor to the success of the models as they had greater training data. The use of additional features is also likely to have improved the FEA model, particularly apparent power and the curtailment classifier which acted as a check on the other model input. We also developed a proxy for wind direction which was novel and attracted the attention of the judging panel.